Deep Learning for High-Frequency Data
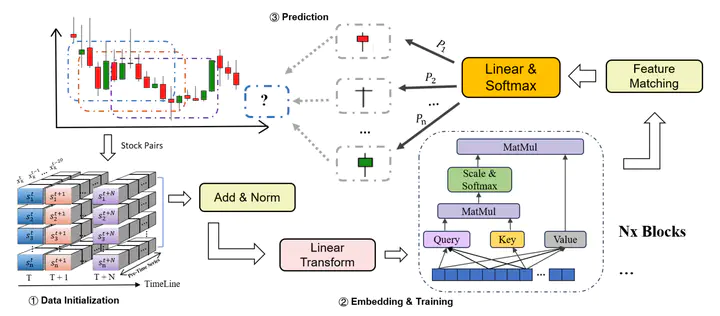
Deep learning has emerged as a powerful tool for analyzing high-frequency financial data, offering sophisticated capabilities to capture complex patterns and nonlinear relationships in market dynamics. These advanced models, particularly those based on Transformer networks, recurrent neural networks (RNNs), and long short-term memory (LSTM) networks, can process massive amounts of time-series data to predict market movements, assess volatility, and automate trading decisions with remarkable precision. The ability to simultaneously analyze historical market data, real-time financial news, and macroeconomic trends allows deep learning algorithms to make split-second trading decisions, making them particularly valuable for high-frequency trading applications. Recent research has demonstrated that deep learning models significantly outperform traditional econometric approaches in tasks such as volatility forecasting and market index prediction, especially when dealing with multivariate high-frequency data from hundreds of financial instruments.